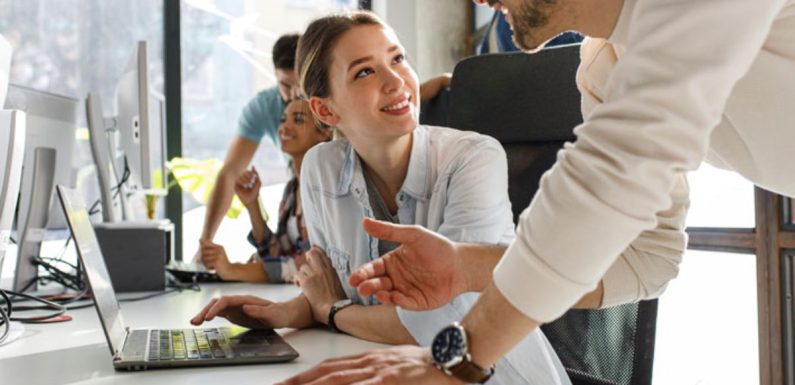
In the realm of artificial intelligence, generative models have garnered significant attention for their ability to create novel content, whether it be text, images, music, or more. These models, fueled by complex algorithms and vast datasets, have exhibited remarkable creativity and potential. However, like any technology, generative AI solutions are not without their pitfalls. This article delves into the common mistakes that often arise when working with generative AI solutions.
By understanding these challenges, developers, researchers, and enthusiasts can navigate the intricacies of generative AI more effectively, ultimately harnessing its power while avoiding potential pitfalls. From biased outputs to ethical concerns, let’s explore the missteps that can occur and how to address them to ensure responsible and beneficial AI innovation.
Mistaking AI as a Magic Wand Without Clear Business Goals:
Generative AI is a powerful tool, but it is not a magic wand. It cannot solve all of your problems. Before you implement a generative AI solution, you need to have clear business goals in mind. What do you hope to achieve with generative AI? Some possible goals include:
- Generate new product ideas
- Improve customer service
- Create more engaging marketing content
- Automate tasks
- Make better decisions
Once you know your goals, you can start to select the right generative AI solution for your needs.
Underestimating the Importance of Data Quality:
Generative AI models are only as good as the data they are trained on. If you use low-quality data, your models will produce low-quality results. This is why it is important to carefully select your data and to clean it thoroughly before training your models.
Here are some tips for ensuring the quality of your data:
- Use data from a variety of sources.
- Remove any incomplete or inaccurate data.
- Standardize the format of your data.
- Label your data carefully.
By taking the time to ensure the quality of your data, you can improve the accuracy and performance of your generative AI models.
Building a Castle in the Sky: Overlooking the Need for a Data Foundation:
A data foundation is a set of data that is used to support all of your business activities. It includes data about your customers, products, and operations. A strong data foundation is essential for generative AI because it provides the models with the information they need to generate accurate and relevant results.
If you overlook the need for a data foundation, your generative AI solutions will be limited in their capabilities. The models will not be able to generate accurate or relevant results, and you will not be able to achieve your business goals.
To build a strong data foundation, you need to carefully collect and curate your data. You need to make sure that the data is clean and accurate, and that it is representative of your target population. You also need to make sure that the data is organized and accessible so that the models can easily access it.
Failing to Monitor and Evaluate Your Models:
Once you have implemented a generative AI solution, it is important to monitor and evaluate its performance. This will help you to identify any areas where the models need improvement. You should also monitor the results of the models to make sure that they are meeting your business goals.
If you fail to monitor and evaluate your models, you will not be able to identify any problems with the models. The models may be generating inaccurate or irrelevant results, and you may not be aware of it. This could lead to you making bad decisions based on the results of the models.
To monitor and evaluate your models, you need to collect data about the performance of the models. This data can include the accuracy of the results, the relevance of the results, and the time it takes the models to generate results. You can then use this data to identify any problems with the models and to make improvements.
By monitoring and evaluating your models, you can ensure that they are performing as expected and that they are meeting your business goals.
Failing to Monitor and Evaluate Your Models:
Once you have implemented a generative AI solution, it is important to monitor and evaluate its performance. This will help you to identify any areas where the models need improvement. You should also monitor the results of the models to make sure that they are meeting your business goals.
There are a number of ways to monitor and evaluate generative AI models. One way is to use metrics such as accuracy, precision, and recall. These metrics can help you to measure how well the models are performing at generating the desired output. You can also use qualitative methods such as user surveys and interviews to get feedback on the models from real users.
By monitoring and evaluating your models, you can identify any areas where they need improvement. You can then make changes to the models or the data they are trained on to improve their performance.
Not being transparent about how generative AI is used:
Generative AI can be used to create content that is indistinguishable from human-generated content. This raises the risk of users being misled about the source of the content. It is important to be transparent about how generative AI is used, and to provide users with information about the limitations of generative AI.
To be transparent about how generative AI is used, you should clearly disclose to users that the content they are seeing is generated by AI. You should also provide information about the limitations of generative AI, such as the fact that the content may not be accurate or complete.
By being transparent about how generative AI is used, you can help to ensure that users are aware of the potential risks and make informed decisions about the content they consume.
Conclusion
Generative AI is a powerful tool that can be used to solve a variety of problems. However, it is important to be aware of the common mistakes that businesses make when implementing generative AI solutions. By avoiding these mistakes, you can increase the chances of success with your generative AI initiatives.