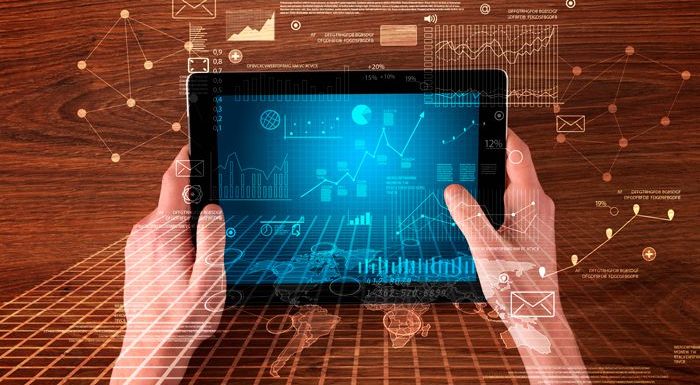
Data is the new oil. Insights derived from massive amounts of information can go beyond planning, budgeting, and forecasting, it can define a brand’s competitive advantage. Big data processed using machine learning can identify emerging opportunities, simulate events and help executives make better business decisions.
Data-driven strategies
In fact, the strategic importance of shifting to data-driven decision making (DDD) is becoming more real. You may have experienced it already without consciously knowing. Remember the time when you visited Amazon, put an item in your cart, changed your mind and eventually abandoned it? Bizarrely, the product you left suddenly keeps popping on every website you visit.
No, that’s not a plain coincidence, and certainly, your mind is not playing tricks on you. That’s what advertisers called retargeting. Through data-driven advertising, potential customers who abandoned their carts are treated as data points in an algorithm that will be targeted to see hyper-relevant offers to close a sale eventually.
The best customer insights
The best customer insights are right under your nose. Social media chats, comments, photos, and videos; corporate presentations and project documents; audit and project completion reports. The insights are all there just waiting to be crunched and interpreted. Take the case of LinkedIn, this data-driven company power up to 300 billion events in which data plays a significant role. Uber is another perfect example. This data-driven tech giant leverages big data to track thousands of cars and drivers and match them up with the best route.
Machine learning’s role
Certainly, data coming from different sources, both structured and unstructured is seemingly impossible to process and much even correlate to derive predictions and insights. This is where machine learning comes in. Big data analyzed through machine learning using products such as WorkFusion Smart Process Automation (SPA) can provide more in-depth customer insights that traditional business intelligence reports cannot provide. Using artificial intelligence, algorithms from SPA can make sense of billions of data regardless of its state or format. This is done through a data mining process that involves data conversion, removing or inferring missing values and data normalization that transforms disparate datasets into a homogenous database. From here, a carefully identified machine learning algorithm from SPA will perform descriptive, predictive and prescriptive analysis, providing customer insights that are deeply buried for years.
How to start
Combining big data and machine learning to uncover the best customer insights is done through a six-step process.
Understand the business and your data
Start with understanding the business problem that needs to be solved together with the strengths and limitations of existing data sources. The data scientist’s creativity is vital at this stage, because of the need to carefully transform business problems into data science problems. If done correctly, the business problem can now be analyzed relative to expected value, allowing data scientists to decompose it into data mining tasks.
The second step is understanding the data. Knowing the strengths and limitations of existing data is vital because various datasets can contain varying information and degrees of veracity. It is possible that some records may need to be purchased to solve a business problem, so be prepared for that. Estimating the costs and benefits of each source is another vital phase of combining big data and machine learning.
Prepare the data and identify a suitable machine learning model
Data preparation and modeling comes next. In here, data conversion and normalization are done to combine data from various sources, both structured or unstructured. This data undergoes exploratory data analysis (EDA) to identify the most suitable learning algorithm, data classification patterns, predictions, and clustering.
This collectively defines your machine learning model. Creating a Whitebox model is the best way to show relationships among data and see if the model is indeed reflecting the reality of the system where it came from. As a tip, it is ideal to start with a simple model and gradually mature it as more is learned about the data.
Model Evaluation and deployment
Once the model is set, it needs to be evaluated to gain valid confidence that it satisfies the business goals. Remember, the objective of data science is to create and support DDD, hence performing both qualitative and quantitative assessments are ideal.
For example, model evaluation techniques, such as sensitivity and specificity allows data scientists to know the probability of the model to get true positives and true negatives. In layman’s term, it measures the model’s capability to classify its data appropriately, which is very useful to mature a machine algorithm model.
The last step is deploying the model into the production system. Typically, the model needs to be optimized to fit the production environment to meet performance and compatibility requirements. That’s why data scientists usually start with a prototype and have developers review how it will work once live. Remember, it is a best practice to involve developers early in any big data with machine learning project so that they can act as advisors, providing critical insight to the data science team.
Key takeaway
Combining big data and machine learning is the future of data-driven decision making. To uncover the best customer insights, organizations don’t have to engage market research companies, bring in expensive consultants or purchase industry reports. Everything that they need is buried in those legacy systems, shared folders, social media sites and even the dusty old filing cabinets of the accounting department.
The journey to DDD is not a walk in the park; however, it is critical to start working on it, before your competitor does. Customer insights are not just an expensive commodity; it will define a company’s standing in the market race and its future.