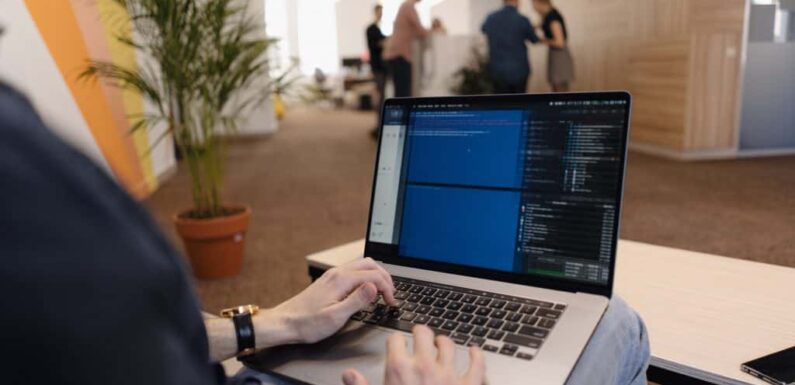
Organizations are moving towards AI-based solutions due to better efficiency, and productivity. AI-based solutions are efficient, quick, read large datasets, and forecast longer.
Humans can’t read such a large amount of data to find hidden patterns. Machine learning-based models can train on large data sets and make accurate predictions. They can find complex hidden patterns in data for strategic decisions.
Ten Steps to Start ML in Business
Starting a machine learning-based solution and selecting an appropriate model is difficult. Machine learning is a complex field. The implementation of ML requires data analysts, software engineers, and IT experts. But, companies like Verta provide ML model management in one place.
This article uses a more straightforward approach for companies to employ ML solutions. However, this approach is good at the experimentation level to be followed. Below, we discuss ten easy steps to follow in implementing ML models in a company.
Step-1 Problem Identification
Machine learning algorithms are not accurate in all situations, depending on many factors. The step involves identifying a business problem that can be solved using ML models. The exact problem, clear goals, processes, options, and expected results are set first.
Step-2 Identify Data Required
Machine learning models are trained on datasets. The performance of machine learning and its capability depends on the dataset. A complete dataset will yield better results than smaller and missing data sets. In the second stage, businesses must know which type of data is required to train an ML model for a problem.
Step-3 Simple is Easy
Keep the first problem small and straightforward to implement. Starting with small problems always helps in experimentation and obtaining results. The ML team would be focused, and changing is easy with different features and parameters.
Step-4 Machine Learning Capacity
There should be a clear picture of the capability and capacity of ML in different problems. Such machine learning models are more accurate in image classification than speech recognition.
Over time, the capability of machine learning is increasing day by day in every field. So the business must have a clear understanding of the ML capabilities in different fields.
Step-5 Data Collection
Data collection is an important step and requires too much care and cautiousness. The machine learning model performance is dependent on this data. The higher and more accurate this data is, the higher the performance of ML results in prediction.
So, the data collected must be complete so that a lot of effort won’t get wasted in finding missing data. Already available data can also be used with new one distributed uniformly.
Step-6 Data Exploration
The data exploration includes the finding of biases in datasets. Biases may influence results at the prediction stage. Like equating males and females to predict male performances compared to females. The bias and variances in the data sets must be addressed at this initial stage.
The selection of features is also involved in data exploration. The choice of features directly affects the performance of the models. The dataset must not have missing values with data normally distributed. So, it is necessary to select optimized features for better working capacity.
Step-7 Data Cleaning
This step involves cleaning data, labeling headers, filling in missing information, and resolving inconsistencies. Besides that, this step requires data segmentation, data flattening, and data imbalance.
The detection of outliers and their treatment are also involved in data cleaning. Further data cleaning also involves labeling data, naming headers, indexing, and treating outliers.
The deletion of missing data causes the unexpected performance of machine learning models. So, it is necessary to avoid deleting missing values, outliers, and wrong entries. They are fixed using different techniques, such as filling data by averaging.
Step-8 Machine learning Model Training
We first equally mixed the datasets to make them uniform at this stage. Datasets are usually divided into three parts: training, cross-validation, and testing. 65% of data is used for training, 20% is used for cross, and 15 % is used for testing.
The efficiency in one part of ML modeling depends on the other. Like good training results lead to good testing results.
Training of the dataset uses cleaned and normalized data for training ML models. ML model training is a long and complex procedure depending on the dataset. Sometimes it takes a minute to train the dataset. But it can also take days to train a machine learning model for better performance.
Step-9 Machine Learning Model Testing
The trained machine learning model is used on the actual system for the solution. Machine learning models are tested in different steps. Change and transformation of features improve the performance of the model. Similarly, parameters are tuned for better output at this stage.
ML model testing is described as performance checks of a trained system. Several different statistics are used to check the accuracy of the model. These statistics include mean squared error, operating curves, and prediction output.
Step-10 Machine Learning Model Evaluation
The machine learning model is evaluated using different metrics. The first evaluation is to check and match the initial goal and objectives. Machine learning models use metrics errors, accuracy percentages, and scores.
This step determines the ML performance is up to the benchmark set in step one or not. Some measures are set in initial stages, and some benchmarks are general.
Final Thought
Incorporating the machine learning model in a traditional business is a complex procedure. Companies are still hesitant to use it for complex business problems. Many ML-based companies are helping with model selection, training, and deployment. These companies follow complex procedures and many stages.
This article provides ten easy steps for starting ML model employment. These essential steps are followed from problem identification to the deployment of the model. Other steps include data collection, data cleaning, model training, and evaluation. Following these steps, businesses can solve ML problems at the initial level. Any business can start using machine learning using the steps discussed above. After successful experimentation, the company can deploy ML solutions at a larger stage.